Assistant Professor Michelle Carey (BSc, PhD, CStat, AdvDSP), holding a BSc in Financial Mathematics from the University of Limerick and a PhD in Statistics awarded by the same institution in 2012, is a member of the School of Mathematics and Statistics at University College Dublin (UCD). She holds credentials as a Chartered Statistician and a certified Advanced Data Science Professional.
Her academic journey began at the University of Limerick, where, after completing her undergraduate and doctoral studies, she served as a Lecturer in Finance at the Kemmy Business School's Department of Accounting and Finance in 2011. In 2013, she embarked on a postdoctoral fellowship in Biostatistics at the Department of Biostatistics and Computational Biology at the University of Rochester School of Medicine and Dentistry in New York, under the mentorship of Professor Hulin Wu, a leading figure in biostatistics and data science. Here, she focused on the evolution of biological systems through differential equations.
In 2015, Michelle was awarded the prestigious Canadian Statistical Sciences Institute Distinguished Post-doctoral Fellowship at McGill University's Department of Mathematics and Statistics in Montreal, Canada. She worked on statistical methods for analyzing functional data, guided by Professor James O. Ramsay, the renowned founder of functional data analysis, and Professor Christian Genest, an authority in multivariate analysis, nonparametric statistics, and extreme-value theory.
Michelle is the Director of the Functional Data Analysis group at University College Dublin.
and is a Deputy-Director responsible for Data and Dynamics at the UCD Centre for Mechanics
Michelle is also a member of:
- UCD Energy Institute,
- Next Generation Energy Systems (NexSys),
- the VistaMilk SFI Research Centre
- the SFI Centre for Research Training in Foundations of Data Science
- the SFI Centre for Research Training in Genomics Data Science
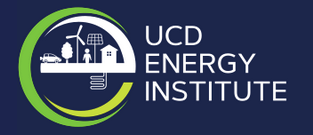
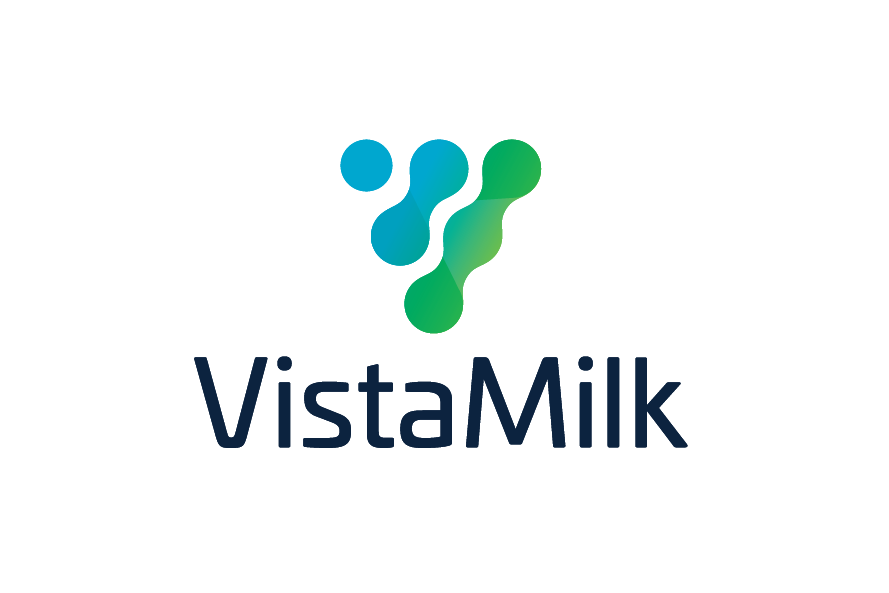
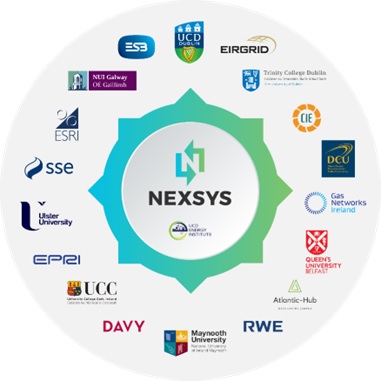

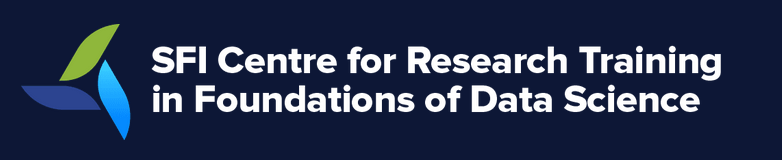